Period of each state
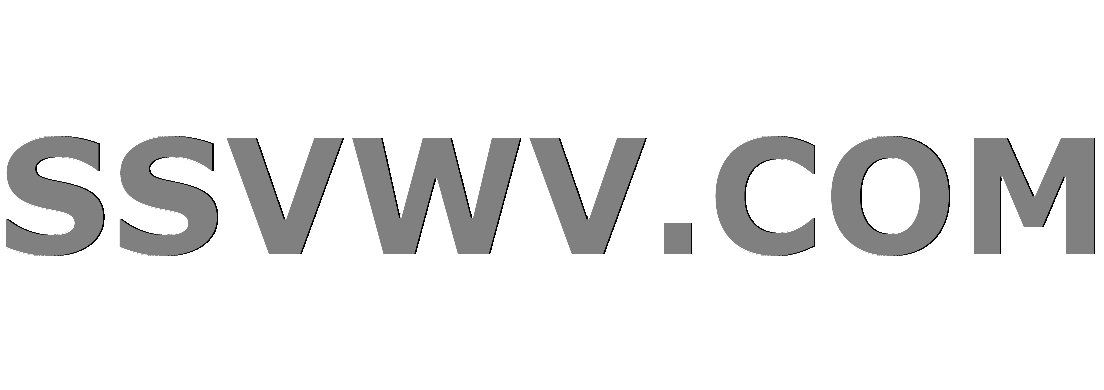
Multi tool use
$begingroup$
I am trying to determine the period of each state $ j = 0, 1, 2$ for this irreducible Markov Chain with transition probability matrix
$$P=begin{bmatrix}0&0&1\1&0&0\frac{1}{2}&frac{1}{2}&0end{bmatrix}$$
All states are of period $1$ because $0 rightarrow 2 rightarrow 0$ and $0 rightarrow 2 rightarrow 1 rightarrow 0$ have $gcd(2,3) =1$
Is this correct and is the proof sound?
probability statistics stochastic-processes markov-chains markov-process
$endgroup$
add a comment |
$begingroup$
I am trying to determine the period of each state $ j = 0, 1, 2$ for this irreducible Markov Chain with transition probability matrix
$$P=begin{bmatrix}0&0&1\1&0&0\frac{1}{2}&frac{1}{2}&0end{bmatrix}$$
All states are of period $1$ because $0 rightarrow 2 rightarrow 0$ and $0 rightarrow 2 rightarrow 1 rightarrow 0$ have $gcd(2,3) =1$
Is this correct and is the proof sound?
probability statistics stochastic-processes markov-chains markov-process
$endgroup$
1
$begingroup$
Yes, the Markov Chain is aperiodic because of the two cycles you have identified whose lengths are relatively prime.
$endgroup$
– Fabio Somenzi
Dec 8 '18 at 6:35
add a comment |
$begingroup$
I am trying to determine the period of each state $ j = 0, 1, 2$ for this irreducible Markov Chain with transition probability matrix
$$P=begin{bmatrix}0&0&1\1&0&0\frac{1}{2}&frac{1}{2}&0end{bmatrix}$$
All states are of period $1$ because $0 rightarrow 2 rightarrow 0$ and $0 rightarrow 2 rightarrow 1 rightarrow 0$ have $gcd(2,3) =1$
Is this correct and is the proof sound?
probability statistics stochastic-processes markov-chains markov-process
$endgroup$
I am trying to determine the period of each state $ j = 0, 1, 2$ for this irreducible Markov Chain with transition probability matrix
$$P=begin{bmatrix}0&0&1\1&0&0\frac{1}{2}&frac{1}{2}&0end{bmatrix}$$
All states are of period $1$ because $0 rightarrow 2 rightarrow 0$ and $0 rightarrow 2 rightarrow 1 rightarrow 0$ have $gcd(2,3) =1$
Is this correct and is the proof sound?
probability statistics stochastic-processes markov-chains markov-process
probability statistics stochastic-processes markov-chains markov-process
asked Dec 8 '18 at 3:28


NoteNote
1468
1468
1
$begingroup$
Yes, the Markov Chain is aperiodic because of the two cycles you have identified whose lengths are relatively prime.
$endgroup$
– Fabio Somenzi
Dec 8 '18 at 6:35
add a comment |
1
$begingroup$
Yes, the Markov Chain is aperiodic because of the two cycles you have identified whose lengths are relatively prime.
$endgroup$
– Fabio Somenzi
Dec 8 '18 at 6:35
1
1
$begingroup$
Yes, the Markov Chain is aperiodic because of the two cycles you have identified whose lengths are relatively prime.
$endgroup$
– Fabio Somenzi
Dec 8 '18 at 6:35
$begingroup$
Yes, the Markov Chain is aperiodic because of the two cycles you have identified whose lengths are relatively prime.
$endgroup$
– Fabio Somenzi
Dec 8 '18 at 6:35
add a comment |
1 Answer
1
active
oldest
votes
$begingroup$
Comment: Here are some computations in R that I have sometimes
found convenient for use with ergodic Markov Chains having small finite
state spaces.
[If the matrix $mathbf{P}$ is based on empirical data, make sure each row of the
transition matrix sums exactly to $0.]$
Establish ergodicity. $mathbf{P}^8$ is a power of the transition matrix in the current Question that
has all positive elements, so the chain is ergodic.
P = matrix(c(0, 0, 1,
1, 0, 0,
.5,.5, 0), nrow=3, byrow=T)
P
[,1] [,2] [,3]
[1,] 0.0 0.0 1
[2,] 1.0 0.0 0
[3,] 0.5 0.5 0
P2 = P %*% P; P4 = P2 %*% P2; P8 = P4 %*% P4; P8
[,1] [,2] [,3]
[1,] 0.4375 0.1875 0.3750
[2,] 0.3750 0.2500 0.3750
[3,] 0.3750 0.1875 0.4375
Compute limiting distribution. The stationary vector $sigma$ with $sigmamathbf{P} = sigma$
is also the limiting distribution of the ergodic chain. For an
ergodic matrix, the left eigenvector with the largest modulus
is real and is proportional to the steady state vector.
eigen(t(P))$vectors # transpose to get LEFT eigenvectors
[,1] [,2] [,3]
[1,] -0.6666667+0i -0.3535534+0.3535534i -0.3535534-0.3535534i
[2,] -0.3333333+0i -0.3535534-0.3535534i -0.3535534+0.3535534i
[3,] -0.6666667+0i 0.7071068+0.0000000i 0.7071068+0.0000000i
s = as.numeric(eigen(t(P))$vectors[,1]); s = s/sum(s); s
[1] 0.4 0.2 0.4 # first-listed vector has largest modulus
s %*% P # to verify stationarity
[,1] [,2] [,3]
[1,] 0.4 0.2 0.4
So $sigma = (.4, .2, .4)$ is the limiting distribution.
Perhaps there is more-elegant R code for this. If so, suggestions are
welcome.
$endgroup$
add a comment |
Your Answer
StackExchange.ifUsing("editor", function () {
return StackExchange.using("mathjaxEditing", function () {
StackExchange.MarkdownEditor.creationCallbacks.add(function (editor, postfix) {
StackExchange.mathjaxEditing.prepareWmdForMathJax(editor, postfix, [["$", "$"], ["\\(","\\)"]]);
});
});
}, "mathjax-editing");
StackExchange.ready(function() {
var channelOptions = {
tags: "".split(" "),
id: "69"
};
initTagRenderer("".split(" "), "".split(" "), channelOptions);
StackExchange.using("externalEditor", function() {
// Have to fire editor after snippets, if snippets enabled
if (StackExchange.settings.snippets.snippetsEnabled) {
StackExchange.using("snippets", function() {
createEditor();
});
}
else {
createEditor();
}
});
function createEditor() {
StackExchange.prepareEditor({
heartbeatType: 'answer',
autoActivateHeartbeat: false,
convertImagesToLinks: true,
noModals: true,
showLowRepImageUploadWarning: true,
reputationToPostImages: 10,
bindNavPrevention: true,
postfix: "",
imageUploader: {
brandingHtml: "Powered by u003ca class="icon-imgur-white" href="https://imgur.com/"u003eu003c/au003e",
contentPolicyHtml: "User contributions licensed under u003ca href="https://creativecommons.org/licenses/by-sa/3.0/"u003ecc by-sa 3.0 with attribution requiredu003c/au003e u003ca href="https://stackoverflow.com/legal/content-policy"u003e(content policy)u003c/au003e",
allowUrls: true
},
noCode: true, onDemand: true,
discardSelector: ".discard-answer"
,immediatelyShowMarkdownHelp:true
});
}
});
Sign up or log in
StackExchange.ready(function () {
StackExchange.helpers.onClickDraftSave('#login-link');
});
Sign up using Google
Sign up using Facebook
Sign up using Email and Password
Post as a guest
Required, but never shown
StackExchange.ready(
function () {
StackExchange.openid.initPostLogin('.new-post-login', 'https%3a%2f%2fmath.stackexchange.com%2fquestions%2f3030667%2fperiod-of-each-state%23new-answer', 'question_page');
}
);
Post as a guest
Required, but never shown
1 Answer
1
active
oldest
votes
1 Answer
1
active
oldest
votes
active
oldest
votes
active
oldest
votes
$begingroup$
Comment: Here are some computations in R that I have sometimes
found convenient for use with ergodic Markov Chains having small finite
state spaces.
[If the matrix $mathbf{P}$ is based on empirical data, make sure each row of the
transition matrix sums exactly to $0.]$
Establish ergodicity. $mathbf{P}^8$ is a power of the transition matrix in the current Question that
has all positive elements, so the chain is ergodic.
P = matrix(c(0, 0, 1,
1, 0, 0,
.5,.5, 0), nrow=3, byrow=T)
P
[,1] [,2] [,3]
[1,] 0.0 0.0 1
[2,] 1.0 0.0 0
[3,] 0.5 0.5 0
P2 = P %*% P; P4 = P2 %*% P2; P8 = P4 %*% P4; P8
[,1] [,2] [,3]
[1,] 0.4375 0.1875 0.3750
[2,] 0.3750 0.2500 0.3750
[3,] 0.3750 0.1875 0.4375
Compute limiting distribution. The stationary vector $sigma$ with $sigmamathbf{P} = sigma$
is also the limiting distribution of the ergodic chain. For an
ergodic matrix, the left eigenvector with the largest modulus
is real and is proportional to the steady state vector.
eigen(t(P))$vectors # transpose to get LEFT eigenvectors
[,1] [,2] [,3]
[1,] -0.6666667+0i -0.3535534+0.3535534i -0.3535534-0.3535534i
[2,] -0.3333333+0i -0.3535534-0.3535534i -0.3535534+0.3535534i
[3,] -0.6666667+0i 0.7071068+0.0000000i 0.7071068+0.0000000i
s = as.numeric(eigen(t(P))$vectors[,1]); s = s/sum(s); s
[1] 0.4 0.2 0.4 # first-listed vector has largest modulus
s %*% P # to verify stationarity
[,1] [,2] [,3]
[1,] 0.4 0.2 0.4
So $sigma = (.4, .2, .4)$ is the limiting distribution.
Perhaps there is more-elegant R code for this. If so, suggestions are
welcome.
$endgroup$
add a comment |
$begingroup$
Comment: Here are some computations in R that I have sometimes
found convenient for use with ergodic Markov Chains having small finite
state spaces.
[If the matrix $mathbf{P}$ is based on empirical data, make sure each row of the
transition matrix sums exactly to $0.]$
Establish ergodicity. $mathbf{P}^8$ is a power of the transition matrix in the current Question that
has all positive elements, so the chain is ergodic.
P = matrix(c(0, 0, 1,
1, 0, 0,
.5,.5, 0), nrow=3, byrow=T)
P
[,1] [,2] [,3]
[1,] 0.0 0.0 1
[2,] 1.0 0.0 0
[3,] 0.5 0.5 0
P2 = P %*% P; P4 = P2 %*% P2; P8 = P4 %*% P4; P8
[,1] [,2] [,3]
[1,] 0.4375 0.1875 0.3750
[2,] 0.3750 0.2500 0.3750
[3,] 0.3750 0.1875 0.4375
Compute limiting distribution. The stationary vector $sigma$ with $sigmamathbf{P} = sigma$
is also the limiting distribution of the ergodic chain. For an
ergodic matrix, the left eigenvector with the largest modulus
is real and is proportional to the steady state vector.
eigen(t(P))$vectors # transpose to get LEFT eigenvectors
[,1] [,2] [,3]
[1,] -0.6666667+0i -0.3535534+0.3535534i -0.3535534-0.3535534i
[2,] -0.3333333+0i -0.3535534-0.3535534i -0.3535534+0.3535534i
[3,] -0.6666667+0i 0.7071068+0.0000000i 0.7071068+0.0000000i
s = as.numeric(eigen(t(P))$vectors[,1]); s = s/sum(s); s
[1] 0.4 0.2 0.4 # first-listed vector has largest modulus
s %*% P # to verify stationarity
[,1] [,2] [,3]
[1,] 0.4 0.2 0.4
So $sigma = (.4, .2, .4)$ is the limiting distribution.
Perhaps there is more-elegant R code for this. If so, suggestions are
welcome.
$endgroup$
add a comment |
$begingroup$
Comment: Here are some computations in R that I have sometimes
found convenient for use with ergodic Markov Chains having small finite
state spaces.
[If the matrix $mathbf{P}$ is based on empirical data, make sure each row of the
transition matrix sums exactly to $0.]$
Establish ergodicity. $mathbf{P}^8$ is a power of the transition matrix in the current Question that
has all positive elements, so the chain is ergodic.
P = matrix(c(0, 0, 1,
1, 0, 0,
.5,.5, 0), nrow=3, byrow=T)
P
[,1] [,2] [,3]
[1,] 0.0 0.0 1
[2,] 1.0 0.0 0
[3,] 0.5 0.5 0
P2 = P %*% P; P4 = P2 %*% P2; P8 = P4 %*% P4; P8
[,1] [,2] [,3]
[1,] 0.4375 0.1875 0.3750
[2,] 0.3750 0.2500 0.3750
[3,] 0.3750 0.1875 0.4375
Compute limiting distribution. The stationary vector $sigma$ with $sigmamathbf{P} = sigma$
is also the limiting distribution of the ergodic chain. For an
ergodic matrix, the left eigenvector with the largest modulus
is real and is proportional to the steady state vector.
eigen(t(P))$vectors # transpose to get LEFT eigenvectors
[,1] [,2] [,3]
[1,] -0.6666667+0i -0.3535534+0.3535534i -0.3535534-0.3535534i
[2,] -0.3333333+0i -0.3535534-0.3535534i -0.3535534+0.3535534i
[3,] -0.6666667+0i 0.7071068+0.0000000i 0.7071068+0.0000000i
s = as.numeric(eigen(t(P))$vectors[,1]); s = s/sum(s); s
[1] 0.4 0.2 0.4 # first-listed vector has largest modulus
s %*% P # to verify stationarity
[,1] [,2] [,3]
[1,] 0.4 0.2 0.4
So $sigma = (.4, .2, .4)$ is the limiting distribution.
Perhaps there is more-elegant R code for this. If so, suggestions are
welcome.
$endgroup$
Comment: Here are some computations in R that I have sometimes
found convenient for use with ergodic Markov Chains having small finite
state spaces.
[If the matrix $mathbf{P}$ is based on empirical data, make sure each row of the
transition matrix sums exactly to $0.]$
Establish ergodicity. $mathbf{P}^8$ is a power of the transition matrix in the current Question that
has all positive elements, so the chain is ergodic.
P = matrix(c(0, 0, 1,
1, 0, 0,
.5,.5, 0), nrow=3, byrow=T)
P
[,1] [,2] [,3]
[1,] 0.0 0.0 1
[2,] 1.0 0.0 0
[3,] 0.5 0.5 0
P2 = P %*% P; P4 = P2 %*% P2; P8 = P4 %*% P4; P8
[,1] [,2] [,3]
[1,] 0.4375 0.1875 0.3750
[2,] 0.3750 0.2500 0.3750
[3,] 0.3750 0.1875 0.4375
Compute limiting distribution. The stationary vector $sigma$ with $sigmamathbf{P} = sigma$
is also the limiting distribution of the ergodic chain. For an
ergodic matrix, the left eigenvector with the largest modulus
is real and is proportional to the steady state vector.
eigen(t(P))$vectors # transpose to get LEFT eigenvectors
[,1] [,2] [,3]
[1,] -0.6666667+0i -0.3535534+0.3535534i -0.3535534-0.3535534i
[2,] -0.3333333+0i -0.3535534-0.3535534i -0.3535534+0.3535534i
[3,] -0.6666667+0i 0.7071068+0.0000000i 0.7071068+0.0000000i
s = as.numeric(eigen(t(P))$vectors[,1]); s = s/sum(s); s
[1] 0.4 0.2 0.4 # first-listed vector has largest modulus
s %*% P # to verify stationarity
[,1] [,2] [,3]
[1,] 0.4 0.2 0.4
So $sigma = (.4, .2, .4)$ is the limiting distribution.
Perhaps there is more-elegant R code for this. If so, suggestions are
welcome.
edited Dec 8 '18 at 8:36
answered Dec 8 '18 at 8:30
BruceETBruceET
35.3k71440
35.3k71440
add a comment |
add a comment |
Thanks for contributing an answer to Mathematics Stack Exchange!
- Please be sure to answer the question. Provide details and share your research!
But avoid …
- Asking for help, clarification, or responding to other answers.
- Making statements based on opinion; back them up with references or personal experience.
Use MathJax to format equations. MathJax reference.
To learn more, see our tips on writing great answers.
Sign up or log in
StackExchange.ready(function () {
StackExchange.helpers.onClickDraftSave('#login-link');
});
Sign up using Google
Sign up using Facebook
Sign up using Email and Password
Post as a guest
Required, but never shown
StackExchange.ready(
function () {
StackExchange.openid.initPostLogin('.new-post-login', 'https%3a%2f%2fmath.stackexchange.com%2fquestions%2f3030667%2fperiod-of-each-state%23new-answer', 'question_page');
}
);
Post as a guest
Required, but never shown
Sign up or log in
StackExchange.ready(function () {
StackExchange.helpers.onClickDraftSave('#login-link');
});
Sign up using Google
Sign up using Facebook
Sign up using Email and Password
Post as a guest
Required, but never shown
Sign up or log in
StackExchange.ready(function () {
StackExchange.helpers.onClickDraftSave('#login-link');
});
Sign up using Google
Sign up using Facebook
Sign up using Email and Password
Post as a guest
Required, but never shown
Sign up or log in
StackExchange.ready(function () {
StackExchange.helpers.onClickDraftSave('#login-link');
});
Sign up using Google
Sign up using Facebook
Sign up using Email and Password
Sign up using Google
Sign up using Facebook
Sign up using Email and Password
Post as a guest
Required, but never shown
Required, but never shown
Required, but never shown
Required, but never shown
Required, but never shown
Required, but never shown
Required, but never shown
Required, but never shown
Required, but never shown
wIKTfcZl3B
1
$begingroup$
Yes, the Markov Chain is aperiodic because of the two cycles you have identified whose lengths are relatively prime.
$endgroup$
– Fabio Somenzi
Dec 8 '18 at 6:35