Equivalent formulation of LASSO?
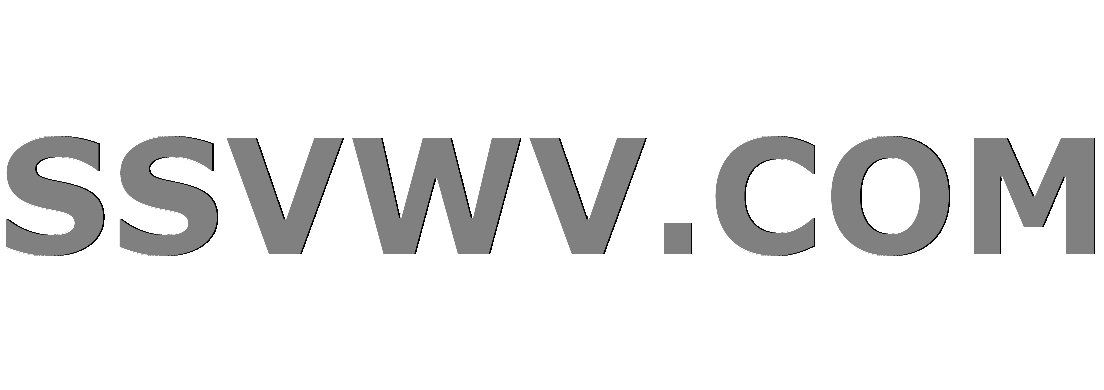
Multi tool use
$begingroup$
I am currently trying to tell wheter or not those two problems are equivalent :
$$min_x |x|_1 text { s.t. } |Ax-y|^2_2 le varepsilon.$$
And
$$min_x |Ax-y|^2_2 text { s.t. } |x|_1le t.$$
With $x$ and $y$ vectors and $A$ a compatible matrix.
I know that the second problem is equivalent to :
$$min_x |Ax-y|^2_2 + lambda |x|_1.$$
(Lagrangian form)
The same process for the first problem would give :
$$min_x |x|_1 + mu |Ax-y|^2_2$$
Does this lead somewhere ?
Thank for your help.
optimization linear-regression
$endgroup$
add a comment |
$begingroup$
I am currently trying to tell wheter or not those two problems are equivalent :
$$min_x |x|_1 text { s.t. } |Ax-y|^2_2 le varepsilon.$$
And
$$min_x |Ax-y|^2_2 text { s.t. } |x|_1le t.$$
With $x$ and $y$ vectors and $A$ a compatible matrix.
I know that the second problem is equivalent to :
$$min_x |Ax-y|^2_2 + lambda |x|_1.$$
(Lagrangian form)
The same process for the first problem would give :
$$min_x |x|_1 + mu |Ax-y|^2_2$$
Does this lead somewhere ?
Thank for your help.
optimization linear-regression
$endgroup$
add a comment |
$begingroup$
I am currently trying to tell wheter or not those two problems are equivalent :
$$min_x |x|_1 text { s.t. } |Ax-y|^2_2 le varepsilon.$$
And
$$min_x |Ax-y|^2_2 text { s.t. } |x|_1le t.$$
With $x$ and $y$ vectors and $A$ a compatible matrix.
I know that the second problem is equivalent to :
$$min_x |Ax-y|^2_2 + lambda |x|_1.$$
(Lagrangian form)
The same process for the first problem would give :
$$min_x |x|_1 + mu |Ax-y|^2_2$$
Does this lead somewhere ?
Thank for your help.
optimization linear-regression
$endgroup$
I am currently trying to tell wheter or not those two problems are equivalent :
$$min_x |x|_1 text { s.t. } |Ax-y|^2_2 le varepsilon.$$
And
$$min_x |Ax-y|^2_2 text { s.t. } |x|_1le t.$$
With $x$ and $y$ vectors and $A$ a compatible matrix.
I know that the second problem is equivalent to :
$$min_x |Ax-y|^2_2 + lambda |x|_1.$$
(Lagrangian form)
The same process for the first problem would give :
$$min_x |x|_1 + mu |Ax-y|^2_2$$
Does this lead somewhere ?
Thank for your help.
optimization linear-regression
optimization linear-regression
edited Dec 20 '18 at 10:05
nicomezi
asked Dec 20 '18 at 9:44
nicomezinicomezi
4,1591920
4,1591920
add a comment |
add a comment |
1 Answer
1
active
oldest
votes
$begingroup$
The first problem
$$min_x |x|_1 text { subject to } |Ax-y|^2_2 le epsilon$$
can be described by the equivalent saddle point problem:
$$
min_xmax_{lambdage 0}left[ |x|_1 +lambda(|Ax-y|_2^2-epsilon)right]=max_{lambdage 0}min_xleft[ |x|_1 +lambda(|Ax-y|_2^2-epsilon)right],
$$ by Lagrange's multiplier theorem. Let $lambda_0ge 0$ be the solution of the above problem such that
$$
max_{lambdage 0}min_xleft[ |x|_1 +lambda(|Ax-y|_2^2-epsilon)right]=min_xleft[ |x|_1 +lambda_0(|Ax-y|_2^2-epsilon)right].
$$If we have $lambda_0 = 0$, then the minimizer should be $x=0$ and $|y|^2_2leqepsilon.$ Let us exclude this trivial case and assume that $lambda_0>0$. By complementary slackness, we should have $|Ax_0-y|_2^2 =epsilon$ where $x_0$ is the solution of the first problem.
In the same manner, the second problem can be equivalently described as
$$
min_{x'}max_{muge 0}left[ |Ax'-y|_2^2 +mu (|x'|_1-t)right]=max_{muge 0}min_{x'}left[ |Ax'-y|_2^2 +mu (|x'|_1-t)right]quadcdots(*),
$$ where $t = |x_0|_1$. If we look at the formulation of the second problem carefully, we can see that the solution $(mu_0, x_0')$ should be $(frac{1}{lambda_0},x_0)$. To see this, one can plug $mu=lambda_0^{-1}$ and $x_0'=x_0$ into the $(*)$ to check if equality holds. In fact, equality holds since given $mu=lambda_0^{-1}$, $x=x_0$ is the minimizer and conversely, given $x=x_0$, $mu =lambda_0^{-1}$ is the maximizer. This establishes that Lagrangian dual problems in this case (in fact, generally) are equivalent.
$endgroup$
$begingroup$
Thanks a lot. Not sure I fully understand your answer for now but all that I get seems very logical and meaningfull. I will look further into it.
$endgroup$
– nicomezi
Dec 20 '18 at 10:50
add a comment |
Your Answer
StackExchange.ifUsing("editor", function () {
return StackExchange.using("mathjaxEditing", function () {
StackExchange.MarkdownEditor.creationCallbacks.add(function (editor, postfix) {
StackExchange.mathjaxEditing.prepareWmdForMathJax(editor, postfix, [["$", "$"], ["\\(","\\)"]]);
});
});
}, "mathjax-editing");
StackExchange.ready(function() {
var channelOptions = {
tags: "".split(" "),
id: "69"
};
initTagRenderer("".split(" "), "".split(" "), channelOptions);
StackExchange.using("externalEditor", function() {
// Have to fire editor after snippets, if snippets enabled
if (StackExchange.settings.snippets.snippetsEnabled) {
StackExchange.using("snippets", function() {
createEditor();
});
}
else {
createEditor();
}
});
function createEditor() {
StackExchange.prepareEditor({
heartbeatType: 'answer',
autoActivateHeartbeat: false,
convertImagesToLinks: true,
noModals: true,
showLowRepImageUploadWarning: true,
reputationToPostImages: 10,
bindNavPrevention: true,
postfix: "",
imageUploader: {
brandingHtml: "Powered by u003ca class="icon-imgur-white" href="https://imgur.com/"u003eu003c/au003e",
contentPolicyHtml: "User contributions licensed under u003ca href="https://creativecommons.org/licenses/by-sa/3.0/"u003ecc by-sa 3.0 with attribution requiredu003c/au003e u003ca href="https://stackoverflow.com/legal/content-policy"u003e(content policy)u003c/au003e",
allowUrls: true
},
noCode: true, onDemand: true,
discardSelector: ".discard-answer"
,immediatelyShowMarkdownHelp:true
});
}
});
Sign up or log in
StackExchange.ready(function () {
StackExchange.helpers.onClickDraftSave('#login-link');
});
Sign up using Google
Sign up using Facebook
Sign up using Email and Password
Post as a guest
Required, but never shown
StackExchange.ready(
function () {
StackExchange.openid.initPostLogin('.new-post-login', 'https%3a%2f%2fmath.stackexchange.com%2fquestions%2f3047347%2fequivalent-formulation-of-lasso%23new-answer', 'question_page');
}
);
Post as a guest
Required, but never shown
1 Answer
1
active
oldest
votes
1 Answer
1
active
oldest
votes
active
oldest
votes
active
oldest
votes
$begingroup$
The first problem
$$min_x |x|_1 text { subject to } |Ax-y|^2_2 le epsilon$$
can be described by the equivalent saddle point problem:
$$
min_xmax_{lambdage 0}left[ |x|_1 +lambda(|Ax-y|_2^2-epsilon)right]=max_{lambdage 0}min_xleft[ |x|_1 +lambda(|Ax-y|_2^2-epsilon)right],
$$ by Lagrange's multiplier theorem. Let $lambda_0ge 0$ be the solution of the above problem such that
$$
max_{lambdage 0}min_xleft[ |x|_1 +lambda(|Ax-y|_2^2-epsilon)right]=min_xleft[ |x|_1 +lambda_0(|Ax-y|_2^2-epsilon)right].
$$If we have $lambda_0 = 0$, then the minimizer should be $x=0$ and $|y|^2_2leqepsilon.$ Let us exclude this trivial case and assume that $lambda_0>0$. By complementary slackness, we should have $|Ax_0-y|_2^2 =epsilon$ where $x_0$ is the solution of the first problem.
In the same manner, the second problem can be equivalently described as
$$
min_{x'}max_{muge 0}left[ |Ax'-y|_2^2 +mu (|x'|_1-t)right]=max_{muge 0}min_{x'}left[ |Ax'-y|_2^2 +mu (|x'|_1-t)right]quadcdots(*),
$$ where $t = |x_0|_1$. If we look at the formulation of the second problem carefully, we can see that the solution $(mu_0, x_0')$ should be $(frac{1}{lambda_0},x_0)$. To see this, one can plug $mu=lambda_0^{-1}$ and $x_0'=x_0$ into the $(*)$ to check if equality holds. In fact, equality holds since given $mu=lambda_0^{-1}$, $x=x_0$ is the minimizer and conversely, given $x=x_0$, $mu =lambda_0^{-1}$ is the maximizer. This establishes that Lagrangian dual problems in this case (in fact, generally) are equivalent.
$endgroup$
$begingroup$
Thanks a lot. Not sure I fully understand your answer for now but all that I get seems very logical and meaningfull. I will look further into it.
$endgroup$
– nicomezi
Dec 20 '18 at 10:50
add a comment |
$begingroup$
The first problem
$$min_x |x|_1 text { subject to } |Ax-y|^2_2 le epsilon$$
can be described by the equivalent saddle point problem:
$$
min_xmax_{lambdage 0}left[ |x|_1 +lambda(|Ax-y|_2^2-epsilon)right]=max_{lambdage 0}min_xleft[ |x|_1 +lambda(|Ax-y|_2^2-epsilon)right],
$$ by Lagrange's multiplier theorem. Let $lambda_0ge 0$ be the solution of the above problem such that
$$
max_{lambdage 0}min_xleft[ |x|_1 +lambda(|Ax-y|_2^2-epsilon)right]=min_xleft[ |x|_1 +lambda_0(|Ax-y|_2^2-epsilon)right].
$$If we have $lambda_0 = 0$, then the minimizer should be $x=0$ and $|y|^2_2leqepsilon.$ Let us exclude this trivial case and assume that $lambda_0>0$. By complementary slackness, we should have $|Ax_0-y|_2^2 =epsilon$ where $x_0$ is the solution of the first problem.
In the same manner, the second problem can be equivalently described as
$$
min_{x'}max_{muge 0}left[ |Ax'-y|_2^2 +mu (|x'|_1-t)right]=max_{muge 0}min_{x'}left[ |Ax'-y|_2^2 +mu (|x'|_1-t)right]quadcdots(*),
$$ where $t = |x_0|_1$. If we look at the formulation of the second problem carefully, we can see that the solution $(mu_0, x_0')$ should be $(frac{1}{lambda_0},x_0)$. To see this, one can plug $mu=lambda_0^{-1}$ and $x_0'=x_0$ into the $(*)$ to check if equality holds. In fact, equality holds since given $mu=lambda_0^{-1}$, $x=x_0$ is the minimizer and conversely, given $x=x_0$, $mu =lambda_0^{-1}$ is the maximizer. This establishes that Lagrangian dual problems in this case (in fact, generally) are equivalent.
$endgroup$
$begingroup$
Thanks a lot. Not sure I fully understand your answer for now but all that I get seems very logical and meaningfull. I will look further into it.
$endgroup$
– nicomezi
Dec 20 '18 at 10:50
add a comment |
$begingroup$
The first problem
$$min_x |x|_1 text { subject to } |Ax-y|^2_2 le epsilon$$
can be described by the equivalent saddle point problem:
$$
min_xmax_{lambdage 0}left[ |x|_1 +lambda(|Ax-y|_2^2-epsilon)right]=max_{lambdage 0}min_xleft[ |x|_1 +lambda(|Ax-y|_2^2-epsilon)right],
$$ by Lagrange's multiplier theorem. Let $lambda_0ge 0$ be the solution of the above problem such that
$$
max_{lambdage 0}min_xleft[ |x|_1 +lambda(|Ax-y|_2^2-epsilon)right]=min_xleft[ |x|_1 +lambda_0(|Ax-y|_2^2-epsilon)right].
$$If we have $lambda_0 = 0$, then the minimizer should be $x=0$ and $|y|^2_2leqepsilon.$ Let us exclude this trivial case and assume that $lambda_0>0$. By complementary slackness, we should have $|Ax_0-y|_2^2 =epsilon$ where $x_0$ is the solution of the first problem.
In the same manner, the second problem can be equivalently described as
$$
min_{x'}max_{muge 0}left[ |Ax'-y|_2^2 +mu (|x'|_1-t)right]=max_{muge 0}min_{x'}left[ |Ax'-y|_2^2 +mu (|x'|_1-t)right]quadcdots(*),
$$ where $t = |x_0|_1$. If we look at the formulation of the second problem carefully, we can see that the solution $(mu_0, x_0')$ should be $(frac{1}{lambda_0},x_0)$. To see this, one can plug $mu=lambda_0^{-1}$ and $x_0'=x_0$ into the $(*)$ to check if equality holds. In fact, equality holds since given $mu=lambda_0^{-1}$, $x=x_0$ is the minimizer and conversely, given $x=x_0$, $mu =lambda_0^{-1}$ is the maximizer. This establishes that Lagrangian dual problems in this case (in fact, generally) are equivalent.
$endgroup$
The first problem
$$min_x |x|_1 text { subject to } |Ax-y|^2_2 le epsilon$$
can be described by the equivalent saddle point problem:
$$
min_xmax_{lambdage 0}left[ |x|_1 +lambda(|Ax-y|_2^2-epsilon)right]=max_{lambdage 0}min_xleft[ |x|_1 +lambda(|Ax-y|_2^2-epsilon)right],
$$ by Lagrange's multiplier theorem. Let $lambda_0ge 0$ be the solution of the above problem such that
$$
max_{lambdage 0}min_xleft[ |x|_1 +lambda(|Ax-y|_2^2-epsilon)right]=min_xleft[ |x|_1 +lambda_0(|Ax-y|_2^2-epsilon)right].
$$If we have $lambda_0 = 0$, then the minimizer should be $x=0$ and $|y|^2_2leqepsilon.$ Let us exclude this trivial case and assume that $lambda_0>0$. By complementary slackness, we should have $|Ax_0-y|_2^2 =epsilon$ where $x_0$ is the solution of the first problem.
In the same manner, the second problem can be equivalently described as
$$
min_{x'}max_{muge 0}left[ |Ax'-y|_2^2 +mu (|x'|_1-t)right]=max_{muge 0}min_{x'}left[ |Ax'-y|_2^2 +mu (|x'|_1-t)right]quadcdots(*),
$$ where $t = |x_0|_1$. If we look at the formulation of the second problem carefully, we can see that the solution $(mu_0, x_0')$ should be $(frac{1}{lambda_0},x_0)$. To see this, one can plug $mu=lambda_0^{-1}$ and $x_0'=x_0$ into the $(*)$ to check if equality holds. In fact, equality holds since given $mu=lambda_0^{-1}$, $x=x_0$ is the minimizer and conversely, given $x=x_0$, $mu =lambda_0^{-1}$ is the maximizer. This establishes that Lagrangian dual problems in this case (in fact, generally) are equivalent.
answered Dec 20 '18 at 10:38
SongSong
15.4k1736
15.4k1736
$begingroup$
Thanks a lot. Not sure I fully understand your answer for now but all that I get seems very logical and meaningfull. I will look further into it.
$endgroup$
– nicomezi
Dec 20 '18 at 10:50
add a comment |
$begingroup$
Thanks a lot. Not sure I fully understand your answer for now but all that I get seems very logical and meaningfull. I will look further into it.
$endgroup$
– nicomezi
Dec 20 '18 at 10:50
$begingroup$
Thanks a lot. Not sure I fully understand your answer for now but all that I get seems very logical and meaningfull. I will look further into it.
$endgroup$
– nicomezi
Dec 20 '18 at 10:50
$begingroup$
Thanks a lot. Not sure I fully understand your answer for now but all that I get seems very logical and meaningfull. I will look further into it.
$endgroup$
– nicomezi
Dec 20 '18 at 10:50
add a comment |
Thanks for contributing an answer to Mathematics Stack Exchange!
- Please be sure to answer the question. Provide details and share your research!
But avoid …
- Asking for help, clarification, or responding to other answers.
- Making statements based on opinion; back them up with references or personal experience.
Use MathJax to format equations. MathJax reference.
To learn more, see our tips on writing great answers.
Sign up or log in
StackExchange.ready(function () {
StackExchange.helpers.onClickDraftSave('#login-link');
});
Sign up using Google
Sign up using Facebook
Sign up using Email and Password
Post as a guest
Required, but never shown
StackExchange.ready(
function () {
StackExchange.openid.initPostLogin('.new-post-login', 'https%3a%2f%2fmath.stackexchange.com%2fquestions%2f3047347%2fequivalent-formulation-of-lasso%23new-answer', 'question_page');
}
);
Post as a guest
Required, but never shown
Sign up or log in
StackExchange.ready(function () {
StackExchange.helpers.onClickDraftSave('#login-link');
});
Sign up using Google
Sign up using Facebook
Sign up using Email and Password
Post as a guest
Required, but never shown
Sign up or log in
StackExchange.ready(function () {
StackExchange.helpers.onClickDraftSave('#login-link');
});
Sign up using Google
Sign up using Facebook
Sign up using Email and Password
Post as a guest
Required, but never shown
Sign up or log in
StackExchange.ready(function () {
StackExchange.helpers.onClickDraftSave('#login-link');
});
Sign up using Google
Sign up using Facebook
Sign up using Email and Password
Sign up using Google
Sign up using Facebook
Sign up using Email and Password
Post as a guest
Required, but never shown
Required, but never shown
Required, but never shown
Required, but never shown
Required, but never shown
Required, but never shown
Required, but never shown
Required, but never shown
Required, but never shown
jWTt9kd91t7,CCaa4fKI8DRi6sE,ioeUvkUNBgxBag2 a,iNosq3fWbkP743GQ 6E,g,AQl w7h,v KvCLPF1saBP,ozNYLy7qEkT,feD yF