Why the constraints in optimization problems are preferred to be convex?
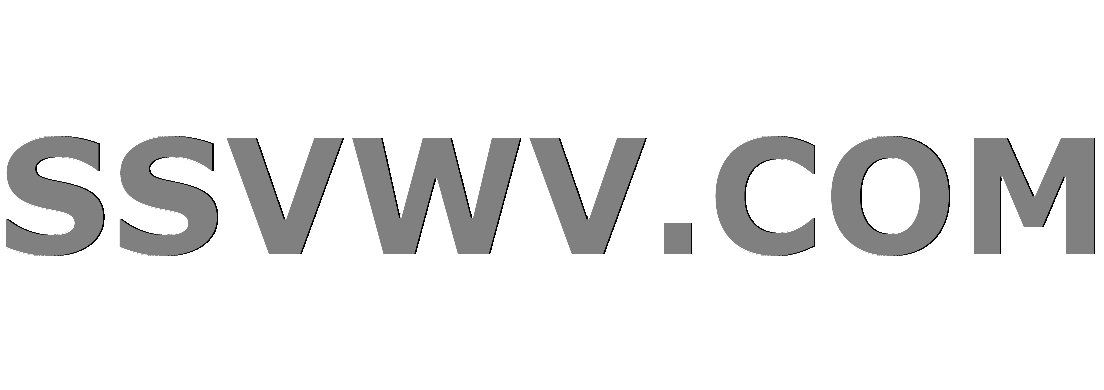
Multi tool use
$begingroup$
Specifically in SVM, it is preferred to have a convex constraint. The preference given to a convex optimization objective is straight-away understandable (to ensure convergence at a global optimum), but it is not quite clear to me why constraints should also be convex.
For eg, in SVM the parameters (weights to the separating hyperplane) are not preferred to be on the surface of a hypersphere. Why?
I read at many places that a non-convex constraint may cause ending up at a local mimima, how is that possible if the objective function is itself convex?
Thank you.
optimization convex-optimization machine-learning non-convex-optimization
$endgroup$
add a comment |
$begingroup$
Specifically in SVM, it is preferred to have a convex constraint. The preference given to a convex optimization objective is straight-away understandable (to ensure convergence at a global optimum), but it is not quite clear to me why constraints should also be convex.
For eg, in SVM the parameters (weights to the separating hyperplane) are not preferred to be on the surface of a hypersphere. Why?
I read at many places that a non-convex constraint may cause ending up at a local mimima, how is that possible if the objective function is itself convex?
Thank you.
optimization convex-optimization machine-learning non-convex-optimization
$endgroup$
add a comment |
$begingroup$
Specifically in SVM, it is preferred to have a convex constraint. The preference given to a convex optimization objective is straight-away understandable (to ensure convergence at a global optimum), but it is not quite clear to me why constraints should also be convex.
For eg, in SVM the parameters (weights to the separating hyperplane) are not preferred to be on the surface of a hypersphere. Why?
I read at many places that a non-convex constraint may cause ending up at a local mimima, how is that possible if the objective function is itself convex?
Thank you.
optimization convex-optimization machine-learning non-convex-optimization
$endgroup$
Specifically in SVM, it is preferred to have a convex constraint. The preference given to a convex optimization objective is straight-away understandable (to ensure convergence at a global optimum), but it is not quite clear to me why constraints should also be convex.
For eg, in SVM the parameters (weights to the separating hyperplane) are not preferred to be on the surface of a hypersphere. Why?
I read at many places that a non-convex constraint may cause ending up at a local mimima, how is that possible if the objective function is itself convex?
Thank you.
optimization convex-optimization machine-learning non-convex-optimization
optimization convex-optimization machine-learning non-convex-optimization
edited Dec 30 '18 at 8:34
Krishna Kumar
asked Dec 30 '18 at 7:32


Krishna KumarKrishna Kumar
32
32
add a comment |
add a comment |
1 Answer
1
active
oldest
votes
$begingroup$
A convex function over a nonconvex domain may have non-global local minima. Just consider to minimize $f(x,y)=x^2 +x +y^2/2$ over the unit circle; you get one non-global minimum.
$endgroup$
$begingroup$
That is my doubt, how can a convex function end up at a local minimum (since the convex function has only one point of minima).
$endgroup$
– Krishna Kumar
Dec 30 '18 at 8:33
$begingroup$
I have an example. Try to work it out and you will see.
$endgroup$
– Dirk
Dec 30 '18 at 8:34
$begingroup$
I worked out the example, found that the point is not the point of global minimum of f. Can you please give some general explanation, or direct me to some resources where I can study this? I searched a lot, but could not find an exhaustive explanation. Thank you.
$endgroup$
– Krishna Kumar
Dec 30 '18 at 8:56
$begingroup$
I am not sure what you mean... If you do some descent algorithm on the constraint problem I gave, you may end up in the non-global minimum. (Oh, and another thing: a convex function can have more than a single minimizer - the set of minimizers can be any convex set.)
$endgroup$
– Dirk
Dec 30 '18 at 8:59
$begingroup$
Guess I got it.. Thank you!
$endgroup$
– Krishna Kumar
Dec 30 '18 at 14:06
|
show 1 more comment
Your Answer
StackExchange.ifUsing("editor", function () {
return StackExchange.using("mathjaxEditing", function () {
StackExchange.MarkdownEditor.creationCallbacks.add(function (editor, postfix) {
StackExchange.mathjaxEditing.prepareWmdForMathJax(editor, postfix, [["$", "$"], ["\\(","\\)"]]);
});
});
}, "mathjax-editing");
StackExchange.ready(function() {
var channelOptions = {
tags: "".split(" "),
id: "69"
};
initTagRenderer("".split(" "), "".split(" "), channelOptions);
StackExchange.using("externalEditor", function() {
// Have to fire editor after snippets, if snippets enabled
if (StackExchange.settings.snippets.snippetsEnabled) {
StackExchange.using("snippets", function() {
createEditor();
});
}
else {
createEditor();
}
});
function createEditor() {
StackExchange.prepareEditor({
heartbeatType: 'answer',
autoActivateHeartbeat: false,
convertImagesToLinks: true,
noModals: true,
showLowRepImageUploadWarning: true,
reputationToPostImages: 10,
bindNavPrevention: true,
postfix: "",
imageUploader: {
brandingHtml: "Powered by u003ca class="icon-imgur-white" href="https://imgur.com/"u003eu003c/au003e",
contentPolicyHtml: "User contributions licensed under u003ca href="https://creativecommons.org/licenses/by-sa/3.0/"u003ecc by-sa 3.0 with attribution requiredu003c/au003e u003ca href="https://stackoverflow.com/legal/content-policy"u003e(content policy)u003c/au003e",
allowUrls: true
},
noCode: true, onDemand: true,
discardSelector: ".discard-answer"
,immediatelyShowMarkdownHelp:true
});
}
});
Sign up or log in
StackExchange.ready(function () {
StackExchange.helpers.onClickDraftSave('#login-link');
});
Sign up using Google
Sign up using Facebook
Sign up using Email and Password
Post as a guest
Required, but never shown
StackExchange.ready(
function () {
StackExchange.openid.initPostLogin('.new-post-login', 'https%3a%2f%2fmath.stackexchange.com%2fquestions%2f3056586%2fwhy-the-constraints-in-optimization-problems-are-preferred-to-be-convex%23new-answer', 'question_page');
}
);
Post as a guest
Required, but never shown
1 Answer
1
active
oldest
votes
1 Answer
1
active
oldest
votes
active
oldest
votes
active
oldest
votes
$begingroup$
A convex function over a nonconvex domain may have non-global local minima. Just consider to minimize $f(x,y)=x^2 +x +y^2/2$ over the unit circle; you get one non-global minimum.
$endgroup$
$begingroup$
That is my doubt, how can a convex function end up at a local minimum (since the convex function has only one point of minima).
$endgroup$
– Krishna Kumar
Dec 30 '18 at 8:33
$begingroup$
I have an example. Try to work it out and you will see.
$endgroup$
– Dirk
Dec 30 '18 at 8:34
$begingroup$
I worked out the example, found that the point is not the point of global minimum of f. Can you please give some general explanation, or direct me to some resources where I can study this? I searched a lot, but could not find an exhaustive explanation. Thank you.
$endgroup$
– Krishna Kumar
Dec 30 '18 at 8:56
$begingroup$
I am not sure what you mean... If you do some descent algorithm on the constraint problem I gave, you may end up in the non-global minimum. (Oh, and another thing: a convex function can have more than a single minimizer - the set of minimizers can be any convex set.)
$endgroup$
– Dirk
Dec 30 '18 at 8:59
$begingroup$
Guess I got it.. Thank you!
$endgroup$
– Krishna Kumar
Dec 30 '18 at 14:06
|
show 1 more comment
$begingroup$
A convex function over a nonconvex domain may have non-global local minima. Just consider to minimize $f(x,y)=x^2 +x +y^2/2$ over the unit circle; you get one non-global minimum.
$endgroup$
$begingroup$
That is my doubt, how can a convex function end up at a local minimum (since the convex function has only one point of minima).
$endgroup$
– Krishna Kumar
Dec 30 '18 at 8:33
$begingroup$
I have an example. Try to work it out and you will see.
$endgroup$
– Dirk
Dec 30 '18 at 8:34
$begingroup$
I worked out the example, found that the point is not the point of global minimum of f. Can you please give some general explanation, or direct me to some resources where I can study this? I searched a lot, but could not find an exhaustive explanation. Thank you.
$endgroup$
– Krishna Kumar
Dec 30 '18 at 8:56
$begingroup$
I am not sure what you mean... If you do some descent algorithm on the constraint problem I gave, you may end up in the non-global minimum. (Oh, and another thing: a convex function can have more than a single minimizer - the set of minimizers can be any convex set.)
$endgroup$
– Dirk
Dec 30 '18 at 8:59
$begingroup$
Guess I got it.. Thank you!
$endgroup$
– Krishna Kumar
Dec 30 '18 at 14:06
|
show 1 more comment
$begingroup$
A convex function over a nonconvex domain may have non-global local minima. Just consider to minimize $f(x,y)=x^2 +x +y^2/2$ over the unit circle; you get one non-global minimum.
$endgroup$
A convex function over a nonconvex domain may have non-global local minima. Just consider to minimize $f(x,y)=x^2 +x +y^2/2$ over the unit circle; you get one non-global minimum.
answered Dec 30 '18 at 8:13


DirkDirk
8,8942447
8,8942447
$begingroup$
That is my doubt, how can a convex function end up at a local minimum (since the convex function has only one point of minima).
$endgroup$
– Krishna Kumar
Dec 30 '18 at 8:33
$begingroup$
I have an example. Try to work it out and you will see.
$endgroup$
– Dirk
Dec 30 '18 at 8:34
$begingroup$
I worked out the example, found that the point is not the point of global minimum of f. Can you please give some general explanation, or direct me to some resources where I can study this? I searched a lot, but could not find an exhaustive explanation. Thank you.
$endgroup$
– Krishna Kumar
Dec 30 '18 at 8:56
$begingroup$
I am not sure what you mean... If you do some descent algorithm on the constraint problem I gave, you may end up in the non-global minimum. (Oh, and another thing: a convex function can have more than a single minimizer - the set of minimizers can be any convex set.)
$endgroup$
– Dirk
Dec 30 '18 at 8:59
$begingroup$
Guess I got it.. Thank you!
$endgroup$
– Krishna Kumar
Dec 30 '18 at 14:06
|
show 1 more comment
$begingroup$
That is my doubt, how can a convex function end up at a local minimum (since the convex function has only one point of minima).
$endgroup$
– Krishna Kumar
Dec 30 '18 at 8:33
$begingroup$
I have an example. Try to work it out and you will see.
$endgroup$
– Dirk
Dec 30 '18 at 8:34
$begingroup$
I worked out the example, found that the point is not the point of global minimum of f. Can you please give some general explanation, or direct me to some resources where I can study this? I searched a lot, but could not find an exhaustive explanation. Thank you.
$endgroup$
– Krishna Kumar
Dec 30 '18 at 8:56
$begingroup$
I am not sure what you mean... If you do some descent algorithm on the constraint problem I gave, you may end up in the non-global minimum. (Oh, and another thing: a convex function can have more than a single minimizer - the set of minimizers can be any convex set.)
$endgroup$
– Dirk
Dec 30 '18 at 8:59
$begingroup$
Guess I got it.. Thank you!
$endgroup$
– Krishna Kumar
Dec 30 '18 at 14:06
$begingroup$
That is my doubt, how can a convex function end up at a local minimum (since the convex function has only one point of minima).
$endgroup$
– Krishna Kumar
Dec 30 '18 at 8:33
$begingroup$
That is my doubt, how can a convex function end up at a local minimum (since the convex function has only one point of minima).
$endgroup$
– Krishna Kumar
Dec 30 '18 at 8:33
$begingroup$
I have an example. Try to work it out and you will see.
$endgroup$
– Dirk
Dec 30 '18 at 8:34
$begingroup$
I have an example. Try to work it out and you will see.
$endgroup$
– Dirk
Dec 30 '18 at 8:34
$begingroup$
I worked out the example, found that the point is not the point of global minimum of f. Can you please give some general explanation, or direct me to some resources where I can study this? I searched a lot, but could not find an exhaustive explanation. Thank you.
$endgroup$
– Krishna Kumar
Dec 30 '18 at 8:56
$begingroup$
I worked out the example, found that the point is not the point of global minimum of f. Can you please give some general explanation, or direct me to some resources where I can study this? I searched a lot, but could not find an exhaustive explanation. Thank you.
$endgroup$
– Krishna Kumar
Dec 30 '18 at 8:56
$begingroup$
I am not sure what you mean... If you do some descent algorithm on the constraint problem I gave, you may end up in the non-global minimum. (Oh, and another thing: a convex function can have more than a single minimizer - the set of minimizers can be any convex set.)
$endgroup$
– Dirk
Dec 30 '18 at 8:59
$begingroup$
I am not sure what you mean... If you do some descent algorithm on the constraint problem I gave, you may end up in the non-global minimum. (Oh, and another thing: a convex function can have more than a single minimizer - the set of minimizers can be any convex set.)
$endgroup$
– Dirk
Dec 30 '18 at 8:59
$begingroup$
Guess I got it.. Thank you!
$endgroup$
– Krishna Kumar
Dec 30 '18 at 14:06
$begingroup$
Guess I got it.. Thank you!
$endgroup$
– Krishna Kumar
Dec 30 '18 at 14:06
|
show 1 more comment
Thanks for contributing an answer to Mathematics Stack Exchange!
- Please be sure to answer the question. Provide details and share your research!
But avoid …
- Asking for help, clarification, or responding to other answers.
- Making statements based on opinion; back them up with references or personal experience.
Use MathJax to format equations. MathJax reference.
To learn more, see our tips on writing great answers.
Sign up or log in
StackExchange.ready(function () {
StackExchange.helpers.onClickDraftSave('#login-link');
});
Sign up using Google
Sign up using Facebook
Sign up using Email and Password
Post as a guest
Required, but never shown
StackExchange.ready(
function () {
StackExchange.openid.initPostLogin('.new-post-login', 'https%3a%2f%2fmath.stackexchange.com%2fquestions%2f3056586%2fwhy-the-constraints-in-optimization-problems-are-preferred-to-be-convex%23new-answer', 'question_page');
}
);
Post as a guest
Required, but never shown
Sign up or log in
StackExchange.ready(function () {
StackExchange.helpers.onClickDraftSave('#login-link');
});
Sign up using Google
Sign up using Facebook
Sign up using Email and Password
Post as a guest
Required, but never shown
Sign up or log in
StackExchange.ready(function () {
StackExchange.helpers.onClickDraftSave('#login-link');
});
Sign up using Google
Sign up using Facebook
Sign up using Email and Password
Post as a guest
Required, but never shown
Sign up or log in
StackExchange.ready(function () {
StackExchange.helpers.onClickDraftSave('#login-link');
});
Sign up using Google
Sign up using Facebook
Sign up using Email and Password
Sign up using Google
Sign up using Facebook
Sign up using Email and Password
Post as a guest
Required, but never shown
Required, but never shown
Required, but never shown
Required, but never shown
Required, but never shown
Required, but never shown
Required, but never shown
Required, but never shown
Required, but never shown
bhKUBOQQz,SiUThzwwWKwI3hkN,TEn,chY 4O8E,75X29g OYhmDaWW1gZj00LEv9mo0QhPfWnd,VJi