Proving $(X,Y)$ is a normal vector when $Xsim N(1,1)$ and $Ymid Xsim N(3X,4)$
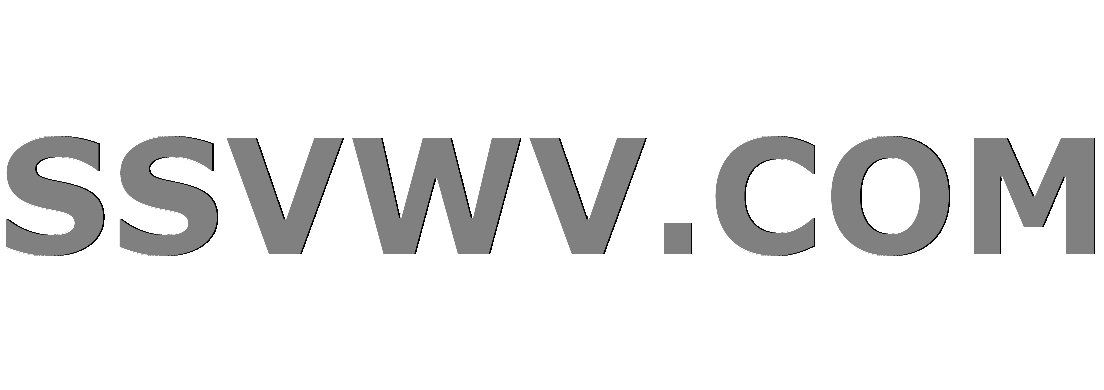
Multi tool use
$begingroup$
Suppose I have a random vector $(X,Y)$ with $Xsimmathcal{N}(1,1)$ and $Y|X = x simmathcal{N}(3x,4)$.
I need to prove that $(X,Y)$ is a normal vector as well.
To do that I want to explicitly write the vector of expected values and the $2x2$ matrix of variance and covariance.
I know that the first entry of the vector of expected values is 1 and the entry $C_{1,1}$ of the matrix is 1 as well. However I am struggling to see how I can derive the density function of Y from the conditional one.
Any suggestions?
probability normal-distribution conditional-probability bivariate-distributions
$endgroup$
add a comment |
$begingroup$
Suppose I have a random vector $(X,Y)$ with $Xsimmathcal{N}(1,1)$ and $Y|X = x simmathcal{N}(3x,4)$.
I need to prove that $(X,Y)$ is a normal vector as well.
To do that I want to explicitly write the vector of expected values and the $2x2$ matrix of variance and covariance.
I know that the first entry of the vector of expected values is 1 and the entry $C_{1,1}$ of the matrix is 1 as well. However I am struggling to see how I can derive the density function of Y from the conditional one.
Any suggestions?
probability normal-distribution conditional-probability bivariate-distributions
$endgroup$
$begingroup$
Joint density of $(X,Y)$ is $f_{X,Y}(x,y)=f_{Ymid X}(y)f_X(x)=...$
$endgroup$
– StubbornAtom
Jan 4 at 15:07
$begingroup$
Yes, but I am trying to calculate E[Y], V[Y] and Cov[X,Y] to "fill" the matrices. I am struggling with Cov[X,Y], specifically with E[XY]. Do you have an hint for that?
$endgroup$
– qcc101
Jan 4 at 15:10
$begingroup$
Comment made by @StubbornAtom below his/her answer needs to be emphasized. Just finding the mean vector and the variance-covariance matrix do not show the joint-normality of $X$ and $Y$.
$endgroup$
– Just_to_Answer
Jan 4 at 20:15
add a comment |
$begingroup$
Suppose I have a random vector $(X,Y)$ with $Xsimmathcal{N}(1,1)$ and $Y|X = x simmathcal{N}(3x,4)$.
I need to prove that $(X,Y)$ is a normal vector as well.
To do that I want to explicitly write the vector of expected values and the $2x2$ matrix of variance and covariance.
I know that the first entry of the vector of expected values is 1 and the entry $C_{1,1}$ of the matrix is 1 as well. However I am struggling to see how I can derive the density function of Y from the conditional one.
Any suggestions?
probability normal-distribution conditional-probability bivariate-distributions
$endgroup$
Suppose I have a random vector $(X,Y)$ with $Xsimmathcal{N}(1,1)$ and $Y|X = x simmathcal{N}(3x,4)$.
I need to prove that $(X,Y)$ is a normal vector as well.
To do that I want to explicitly write the vector of expected values and the $2x2$ matrix of variance and covariance.
I know that the first entry of the vector of expected values is 1 and the entry $C_{1,1}$ of the matrix is 1 as well. However I am struggling to see how I can derive the density function of Y from the conditional one.
Any suggestions?
probability normal-distribution conditional-probability bivariate-distributions
probability normal-distribution conditional-probability bivariate-distributions
edited Jan 4 at 19:02


StubbornAtom
6,29831440
6,29831440
asked Jan 4 at 14:48
qcc101qcc101
629213
629213
$begingroup$
Joint density of $(X,Y)$ is $f_{X,Y}(x,y)=f_{Ymid X}(y)f_X(x)=...$
$endgroup$
– StubbornAtom
Jan 4 at 15:07
$begingroup$
Yes, but I am trying to calculate E[Y], V[Y] and Cov[X,Y] to "fill" the matrices. I am struggling with Cov[X,Y], specifically with E[XY]. Do you have an hint for that?
$endgroup$
– qcc101
Jan 4 at 15:10
$begingroup$
Comment made by @StubbornAtom below his/her answer needs to be emphasized. Just finding the mean vector and the variance-covariance matrix do not show the joint-normality of $X$ and $Y$.
$endgroup$
– Just_to_Answer
Jan 4 at 20:15
add a comment |
$begingroup$
Joint density of $(X,Y)$ is $f_{X,Y}(x,y)=f_{Ymid X}(y)f_X(x)=...$
$endgroup$
– StubbornAtom
Jan 4 at 15:07
$begingroup$
Yes, but I am trying to calculate E[Y], V[Y] and Cov[X,Y] to "fill" the matrices. I am struggling with Cov[X,Y], specifically with E[XY]. Do you have an hint for that?
$endgroup$
– qcc101
Jan 4 at 15:10
$begingroup$
Comment made by @StubbornAtom below his/her answer needs to be emphasized. Just finding the mean vector and the variance-covariance matrix do not show the joint-normality of $X$ and $Y$.
$endgroup$
– Just_to_Answer
Jan 4 at 20:15
$begingroup$
Joint density of $(X,Y)$ is $f_{X,Y}(x,y)=f_{Ymid X}(y)f_X(x)=...$
$endgroup$
– StubbornAtom
Jan 4 at 15:07
$begingroup$
Joint density of $(X,Y)$ is $f_{X,Y}(x,y)=f_{Ymid X}(y)f_X(x)=...$
$endgroup$
– StubbornAtom
Jan 4 at 15:07
$begingroup$
Yes, but I am trying to calculate E[Y], V[Y] and Cov[X,Y] to "fill" the matrices. I am struggling with Cov[X,Y], specifically with E[XY]. Do you have an hint for that?
$endgroup$
– qcc101
Jan 4 at 15:10
$begingroup$
Yes, but I am trying to calculate E[Y], V[Y] and Cov[X,Y] to "fill" the matrices. I am struggling with Cov[X,Y], specifically with E[XY]. Do you have an hint for that?
$endgroup$
– qcc101
Jan 4 at 15:10
$begingroup$
Comment made by @StubbornAtom below his/her answer needs to be emphasized. Just finding the mean vector and the variance-covariance matrix do not show the joint-normality of $X$ and $Y$.
$endgroup$
– Just_to_Answer
Jan 4 at 20:15
$begingroup$
Comment made by @StubbornAtom below his/her answer needs to be emphasized. Just finding the mean vector and the variance-covariance matrix do not show the joint-normality of $X$ and $Y$.
$endgroup$
– Just_to_Answer
Jan 4 at 20:15
add a comment |
2 Answers
2
active
oldest
votes
$begingroup$
You could say $Y=3X+Z$ where $Zsim N(0,4)$ independent of $X$.
It is then an easy step to say that $Ysim N(3times 1+0,3^2times 1+4)$ and
- $E[X]=1$
- $E[Y]=3$
- $text{Var}(X)=1$
- $text{Var}(Y)=13$
- $text{Cov}(X,Y)=text{Cov}(X,3X)=3text{Var}(X)=3$
$endgroup$
$begingroup$
This is the direction I took as well. However I did not use an additional Z. Therefore I am struggling with calculating the covariance between X and Y, could you take a look at my comment to the answer below?
$endgroup$
– qcc101
Jan 4 at 15:28
add a comment |
$begingroup$
You can proceed with moment generating functions.
Joint MGF of $(X,Y)$ is
begin{align}
M(s,t)&=Eleft[e^{sX+tY}right]
\&=Eleft[E(e^{sX+tY}mid X)right]
\&=Eleft[e^{sX}E(e^{tY}mid X)right]
end{align}
From the given information, you should be able to show that this MGF corresponds to the MGF of a bivariate normal distribution. That would complete your proof using the uniqueness property of MGF.
$endgroup$
$begingroup$
I am not sure I am following you. In my problem I did find Variance and Expected value of Y, using the tower property of conditioning. I stil need to find the two symmetric entries of the matrix of variance and covariance to complete what I want to do. That is I need to find Covariance[X,Y]. Calculating, I get to this: $$Cov[X,Y] = E[XY] - 3E[X^2] -E[Y] + 3E[X]$$ Above, I know everything except E[XY]. I did the following: $ E[XY] = E[ E[XY|X]] = E[YE[X|X]] = E[Y E[X]]$ but I get the wrong result ( covariance should be 3 but I get -3). Could you point out what I did wrong?
$endgroup$
– qcc101
Jan 4 at 15:26
$begingroup$
@qcc101 $E(XY)=E(E(XYmid X))=E(XE(Ymid X))=...$
$endgroup$
– StubbornAtom
Jan 4 at 15:29
$begingroup$
Right, silly mistake. I will investigate the part about moment generating functions as well. Thank you.
$endgroup$
– qcc101
Jan 4 at 15:30
1
$begingroup$
@qcc101 Without the argument that $X$ and $Y$ are linear combinations of independent normal variables and hence $(X,Y)$ is bivariate normal (as seen in the answer by @Henry), I don't think finding the variance-covariance matrix (only) as you are trying to do is sufficient for the given question.
$endgroup$
– StubbornAtom
Jan 4 at 15:37
add a comment |
Your Answer
StackExchange.ifUsing("editor", function () {
return StackExchange.using("mathjaxEditing", function () {
StackExchange.MarkdownEditor.creationCallbacks.add(function (editor, postfix) {
StackExchange.mathjaxEditing.prepareWmdForMathJax(editor, postfix, [["$", "$"], ["\\(","\\)"]]);
});
});
}, "mathjax-editing");
StackExchange.ready(function() {
var channelOptions = {
tags: "".split(" "),
id: "69"
};
initTagRenderer("".split(" "), "".split(" "), channelOptions);
StackExchange.using("externalEditor", function() {
// Have to fire editor after snippets, if snippets enabled
if (StackExchange.settings.snippets.snippetsEnabled) {
StackExchange.using("snippets", function() {
createEditor();
});
}
else {
createEditor();
}
});
function createEditor() {
StackExchange.prepareEditor({
heartbeatType: 'answer',
autoActivateHeartbeat: false,
convertImagesToLinks: true,
noModals: true,
showLowRepImageUploadWarning: true,
reputationToPostImages: 10,
bindNavPrevention: true,
postfix: "",
imageUploader: {
brandingHtml: "Powered by u003ca class="icon-imgur-white" href="https://imgur.com/"u003eu003c/au003e",
contentPolicyHtml: "User contributions licensed under u003ca href="https://creativecommons.org/licenses/by-sa/3.0/"u003ecc by-sa 3.0 with attribution requiredu003c/au003e u003ca href="https://stackoverflow.com/legal/content-policy"u003e(content policy)u003c/au003e",
allowUrls: true
},
noCode: true, onDemand: true,
discardSelector: ".discard-answer"
,immediatelyShowMarkdownHelp:true
});
}
});
Sign up or log in
StackExchange.ready(function () {
StackExchange.helpers.onClickDraftSave('#login-link');
});
Sign up using Google
Sign up using Facebook
Sign up using Email and Password
Post as a guest
Required, but never shown
StackExchange.ready(
function () {
StackExchange.openid.initPostLogin('.new-post-login', 'https%3a%2f%2fmath.stackexchange.com%2fquestions%2f3061724%2fproving-x-y-is-a-normal-vector-when-x-sim-n1-1-and-y-mid-x-sim-n3x-4%23new-answer', 'question_page');
}
);
Post as a guest
Required, but never shown
2 Answers
2
active
oldest
votes
2 Answers
2
active
oldest
votes
active
oldest
votes
active
oldest
votes
$begingroup$
You could say $Y=3X+Z$ where $Zsim N(0,4)$ independent of $X$.
It is then an easy step to say that $Ysim N(3times 1+0,3^2times 1+4)$ and
- $E[X]=1$
- $E[Y]=3$
- $text{Var}(X)=1$
- $text{Var}(Y)=13$
- $text{Cov}(X,Y)=text{Cov}(X,3X)=3text{Var}(X)=3$
$endgroup$
$begingroup$
This is the direction I took as well. However I did not use an additional Z. Therefore I am struggling with calculating the covariance between X and Y, could you take a look at my comment to the answer below?
$endgroup$
– qcc101
Jan 4 at 15:28
add a comment |
$begingroup$
You could say $Y=3X+Z$ where $Zsim N(0,4)$ independent of $X$.
It is then an easy step to say that $Ysim N(3times 1+0,3^2times 1+4)$ and
- $E[X]=1$
- $E[Y]=3$
- $text{Var}(X)=1$
- $text{Var}(Y)=13$
- $text{Cov}(X,Y)=text{Cov}(X,3X)=3text{Var}(X)=3$
$endgroup$
$begingroup$
This is the direction I took as well. However I did not use an additional Z. Therefore I am struggling with calculating the covariance between X and Y, could you take a look at my comment to the answer below?
$endgroup$
– qcc101
Jan 4 at 15:28
add a comment |
$begingroup$
You could say $Y=3X+Z$ where $Zsim N(0,4)$ independent of $X$.
It is then an easy step to say that $Ysim N(3times 1+0,3^2times 1+4)$ and
- $E[X]=1$
- $E[Y]=3$
- $text{Var}(X)=1$
- $text{Var}(Y)=13$
- $text{Cov}(X,Y)=text{Cov}(X,3X)=3text{Var}(X)=3$
$endgroup$
You could say $Y=3X+Z$ where $Zsim N(0,4)$ independent of $X$.
It is then an easy step to say that $Ysim N(3times 1+0,3^2times 1+4)$ and
- $E[X]=1$
- $E[Y]=3$
- $text{Var}(X)=1$
- $text{Var}(Y)=13$
- $text{Cov}(X,Y)=text{Cov}(X,3X)=3text{Var}(X)=3$
answered Jan 4 at 15:26
HenryHenry
101k482170
101k482170
$begingroup$
This is the direction I took as well. However I did not use an additional Z. Therefore I am struggling with calculating the covariance between X and Y, could you take a look at my comment to the answer below?
$endgroup$
– qcc101
Jan 4 at 15:28
add a comment |
$begingroup$
This is the direction I took as well. However I did not use an additional Z. Therefore I am struggling with calculating the covariance between X and Y, could you take a look at my comment to the answer below?
$endgroup$
– qcc101
Jan 4 at 15:28
$begingroup$
This is the direction I took as well. However I did not use an additional Z. Therefore I am struggling with calculating the covariance between X and Y, could you take a look at my comment to the answer below?
$endgroup$
– qcc101
Jan 4 at 15:28
$begingroup$
This is the direction I took as well. However I did not use an additional Z. Therefore I am struggling with calculating the covariance between X and Y, could you take a look at my comment to the answer below?
$endgroup$
– qcc101
Jan 4 at 15:28
add a comment |
$begingroup$
You can proceed with moment generating functions.
Joint MGF of $(X,Y)$ is
begin{align}
M(s,t)&=Eleft[e^{sX+tY}right]
\&=Eleft[E(e^{sX+tY}mid X)right]
\&=Eleft[e^{sX}E(e^{tY}mid X)right]
end{align}
From the given information, you should be able to show that this MGF corresponds to the MGF of a bivariate normal distribution. That would complete your proof using the uniqueness property of MGF.
$endgroup$
$begingroup$
I am not sure I am following you. In my problem I did find Variance and Expected value of Y, using the tower property of conditioning. I stil need to find the two symmetric entries of the matrix of variance and covariance to complete what I want to do. That is I need to find Covariance[X,Y]. Calculating, I get to this: $$Cov[X,Y] = E[XY] - 3E[X^2] -E[Y] + 3E[X]$$ Above, I know everything except E[XY]. I did the following: $ E[XY] = E[ E[XY|X]] = E[YE[X|X]] = E[Y E[X]]$ but I get the wrong result ( covariance should be 3 but I get -3). Could you point out what I did wrong?
$endgroup$
– qcc101
Jan 4 at 15:26
$begingroup$
@qcc101 $E(XY)=E(E(XYmid X))=E(XE(Ymid X))=...$
$endgroup$
– StubbornAtom
Jan 4 at 15:29
$begingroup$
Right, silly mistake. I will investigate the part about moment generating functions as well. Thank you.
$endgroup$
– qcc101
Jan 4 at 15:30
1
$begingroup$
@qcc101 Without the argument that $X$ and $Y$ are linear combinations of independent normal variables and hence $(X,Y)$ is bivariate normal (as seen in the answer by @Henry), I don't think finding the variance-covariance matrix (only) as you are trying to do is sufficient for the given question.
$endgroup$
– StubbornAtom
Jan 4 at 15:37
add a comment |
$begingroup$
You can proceed with moment generating functions.
Joint MGF of $(X,Y)$ is
begin{align}
M(s,t)&=Eleft[e^{sX+tY}right]
\&=Eleft[E(e^{sX+tY}mid X)right]
\&=Eleft[e^{sX}E(e^{tY}mid X)right]
end{align}
From the given information, you should be able to show that this MGF corresponds to the MGF of a bivariate normal distribution. That would complete your proof using the uniqueness property of MGF.
$endgroup$
$begingroup$
I am not sure I am following you. In my problem I did find Variance and Expected value of Y, using the tower property of conditioning. I stil need to find the two symmetric entries of the matrix of variance and covariance to complete what I want to do. That is I need to find Covariance[X,Y]. Calculating, I get to this: $$Cov[X,Y] = E[XY] - 3E[X^2] -E[Y] + 3E[X]$$ Above, I know everything except E[XY]. I did the following: $ E[XY] = E[ E[XY|X]] = E[YE[X|X]] = E[Y E[X]]$ but I get the wrong result ( covariance should be 3 but I get -3). Could you point out what I did wrong?
$endgroup$
– qcc101
Jan 4 at 15:26
$begingroup$
@qcc101 $E(XY)=E(E(XYmid X))=E(XE(Ymid X))=...$
$endgroup$
– StubbornAtom
Jan 4 at 15:29
$begingroup$
Right, silly mistake. I will investigate the part about moment generating functions as well. Thank you.
$endgroup$
– qcc101
Jan 4 at 15:30
1
$begingroup$
@qcc101 Without the argument that $X$ and $Y$ are linear combinations of independent normal variables and hence $(X,Y)$ is bivariate normal (as seen in the answer by @Henry), I don't think finding the variance-covariance matrix (only) as you are trying to do is sufficient for the given question.
$endgroup$
– StubbornAtom
Jan 4 at 15:37
add a comment |
$begingroup$
You can proceed with moment generating functions.
Joint MGF of $(X,Y)$ is
begin{align}
M(s,t)&=Eleft[e^{sX+tY}right]
\&=Eleft[E(e^{sX+tY}mid X)right]
\&=Eleft[e^{sX}E(e^{tY}mid X)right]
end{align}
From the given information, you should be able to show that this MGF corresponds to the MGF of a bivariate normal distribution. That would complete your proof using the uniqueness property of MGF.
$endgroup$
You can proceed with moment generating functions.
Joint MGF of $(X,Y)$ is
begin{align}
M(s,t)&=Eleft[e^{sX+tY}right]
\&=Eleft[E(e^{sX+tY}mid X)right]
\&=Eleft[e^{sX}E(e^{tY}mid X)right]
end{align}
From the given information, you should be able to show that this MGF corresponds to the MGF of a bivariate normal distribution. That would complete your proof using the uniqueness property of MGF.
answered Jan 4 at 15:17


StubbornAtomStubbornAtom
6,29831440
6,29831440
$begingroup$
I am not sure I am following you. In my problem I did find Variance and Expected value of Y, using the tower property of conditioning. I stil need to find the two symmetric entries of the matrix of variance and covariance to complete what I want to do. That is I need to find Covariance[X,Y]. Calculating, I get to this: $$Cov[X,Y] = E[XY] - 3E[X^2] -E[Y] + 3E[X]$$ Above, I know everything except E[XY]. I did the following: $ E[XY] = E[ E[XY|X]] = E[YE[X|X]] = E[Y E[X]]$ but I get the wrong result ( covariance should be 3 but I get -3). Could you point out what I did wrong?
$endgroup$
– qcc101
Jan 4 at 15:26
$begingroup$
@qcc101 $E(XY)=E(E(XYmid X))=E(XE(Ymid X))=...$
$endgroup$
– StubbornAtom
Jan 4 at 15:29
$begingroup$
Right, silly mistake. I will investigate the part about moment generating functions as well. Thank you.
$endgroup$
– qcc101
Jan 4 at 15:30
1
$begingroup$
@qcc101 Without the argument that $X$ and $Y$ are linear combinations of independent normal variables and hence $(X,Y)$ is bivariate normal (as seen in the answer by @Henry), I don't think finding the variance-covariance matrix (only) as you are trying to do is sufficient for the given question.
$endgroup$
– StubbornAtom
Jan 4 at 15:37
add a comment |
$begingroup$
I am not sure I am following you. In my problem I did find Variance and Expected value of Y, using the tower property of conditioning. I stil need to find the two symmetric entries of the matrix of variance and covariance to complete what I want to do. That is I need to find Covariance[X,Y]. Calculating, I get to this: $$Cov[X,Y] = E[XY] - 3E[X^2] -E[Y] + 3E[X]$$ Above, I know everything except E[XY]. I did the following: $ E[XY] = E[ E[XY|X]] = E[YE[X|X]] = E[Y E[X]]$ but I get the wrong result ( covariance should be 3 but I get -3). Could you point out what I did wrong?
$endgroup$
– qcc101
Jan 4 at 15:26
$begingroup$
@qcc101 $E(XY)=E(E(XYmid X))=E(XE(Ymid X))=...$
$endgroup$
– StubbornAtom
Jan 4 at 15:29
$begingroup$
Right, silly mistake. I will investigate the part about moment generating functions as well. Thank you.
$endgroup$
– qcc101
Jan 4 at 15:30
1
$begingroup$
@qcc101 Without the argument that $X$ and $Y$ are linear combinations of independent normal variables and hence $(X,Y)$ is bivariate normal (as seen in the answer by @Henry), I don't think finding the variance-covariance matrix (only) as you are trying to do is sufficient for the given question.
$endgroup$
– StubbornAtom
Jan 4 at 15:37
$begingroup$
I am not sure I am following you. In my problem I did find Variance and Expected value of Y, using the tower property of conditioning. I stil need to find the two symmetric entries of the matrix of variance and covariance to complete what I want to do. That is I need to find Covariance[X,Y]. Calculating, I get to this: $$Cov[X,Y] = E[XY] - 3E[X^2] -E[Y] + 3E[X]$$ Above, I know everything except E[XY]. I did the following: $ E[XY] = E[ E[XY|X]] = E[YE[X|X]] = E[Y E[X]]$ but I get the wrong result ( covariance should be 3 but I get -3). Could you point out what I did wrong?
$endgroup$
– qcc101
Jan 4 at 15:26
$begingroup$
I am not sure I am following you. In my problem I did find Variance and Expected value of Y, using the tower property of conditioning. I stil need to find the two symmetric entries of the matrix of variance and covariance to complete what I want to do. That is I need to find Covariance[X,Y]. Calculating, I get to this: $$Cov[X,Y] = E[XY] - 3E[X^2] -E[Y] + 3E[X]$$ Above, I know everything except E[XY]. I did the following: $ E[XY] = E[ E[XY|X]] = E[YE[X|X]] = E[Y E[X]]$ but I get the wrong result ( covariance should be 3 but I get -3). Could you point out what I did wrong?
$endgroup$
– qcc101
Jan 4 at 15:26
$begingroup$
@qcc101 $E(XY)=E(E(XYmid X))=E(XE(Ymid X))=...$
$endgroup$
– StubbornAtom
Jan 4 at 15:29
$begingroup$
@qcc101 $E(XY)=E(E(XYmid X))=E(XE(Ymid X))=...$
$endgroup$
– StubbornAtom
Jan 4 at 15:29
$begingroup$
Right, silly mistake. I will investigate the part about moment generating functions as well. Thank you.
$endgroup$
– qcc101
Jan 4 at 15:30
$begingroup$
Right, silly mistake. I will investigate the part about moment generating functions as well. Thank you.
$endgroup$
– qcc101
Jan 4 at 15:30
1
1
$begingroup$
@qcc101 Without the argument that $X$ and $Y$ are linear combinations of independent normal variables and hence $(X,Y)$ is bivariate normal (as seen in the answer by @Henry), I don't think finding the variance-covariance matrix (only) as you are trying to do is sufficient for the given question.
$endgroup$
– StubbornAtom
Jan 4 at 15:37
$begingroup$
@qcc101 Without the argument that $X$ and $Y$ are linear combinations of independent normal variables and hence $(X,Y)$ is bivariate normal (as seen in the answer by @Henry), I don't think finding the variance-covariance matrix (only) as you are trying to do is sufficient for the given question.
$endgroup$
– StubbornAtom
Jan 4 at 15:37
add a comment |
Thanks for contributing an answer to Mathematics Stack Exchange!
- Please be sure to answer the question. Provide details and share your research!
But avoid …
- Asking for help, clarification, or responding to other answers.
- Making statements based on opinion; back them up with references or personal experience.
Use MathJax to format equations. MathJax reference.
To learn more, see our tips on writing great answers.
Sign up or log in
StackExchange.ready(function () {
StackExchange.helpers.onClickDraftSave('#login-link');
});
Sign up using Google
Sign up using Facebook
Sign up using Email and Password
Post as a guest
Required, but never shown
StackExchange.ready(
function () {
StackExchange.openid.initPostLogin('.new-post-login', 'https%3a%2f%2fmath.stackexchange.com%2fquestions%2f3061724%2fproving-x-y-is-a-normal-vector-when-x-sim-n1-1-and-y-mid-x-sim-n3x-4%23new-answer', 'question_page');
}
);
Post as a guest
Required, but never shown
Sign up or log in
StackExchange.ready(function () {
StackExchange.helpers.onClickDraftSave('#login-link');
});
Sign up using Google
Sign up using Facebook
Sign up using Email and Password
Post as a guest
Required, but never shown
Sign up or log in
StackExchange.ready(function () {
StackExchange.helpers.onClickDraftSave('#login-link');
});
Sign up using Google
Sign up using Facebook
Sign up using Email and Password
Post as a guest
Required, but never shown
Sign up or log in
StackExchange.ready(function () {
StackExchange.helpers.onClickDraftSave('#login-link');
});
Sign up using Google
Sign up using Facebook
Sign up using Email and Password
Sign up using Google
Sign up using Facebook
Sign up using Email and Password
Post as a guest
Required, but never shown
Required, but never shown
Required, but never shown
Required, but never shown
Required, but never shown
Required, but never shown
Required, but never shown
Required, but never shown
Required, but never shown
YIWt6yQ4 M
$begingroup$
Joint density of $(X,Y)$ is $f_{X,Y}(x,y)=f_{Ymid X}(y)f_X(x)=...$
$endgroup$
– StubbornAtom
Jan 4 at 15:07
$begingroup$
Yes, but I am trying to calculate E[Y], V[Y] and Cov[X,Y] to "fill" the matrices. I am struggling with Cov[X,Y], specifically with E[XY]. Do you have an hint for that?
$endgroup$
– qcc101
Jan 4 at 15:10
$begingroup$
Comment made by @StubbornAtom below his/her answer needs to be emphasized. Just finding the mean vector and the variance-covariance matrix do not show the joint-normality of $X$ and $Y$.
$endgroup$
– Just_to_Answer
Jan 4 at 20:15